India’s AI Initiatives: During a board meeting of Reliance Innovation Council several years ago Mukesh Ambani met with Ramakanth Mashelkar for a thought-inducing discussion. The council directs innovative and transformative strategies at India’s leading and most profitable energy-to-retail conglomerate. In an off-hand manner Ambani declared to Mashelkar “We need to skip straight to something.” Mashelkar explains his response to Fortune India about Ambani’s metaphor during their conversation at this time when Mashelkar is 82-years-old and serves as chairman of the Reliance New Energy Council and chancellor of Jio Institute.
Ambani received advice from Mashelkar that frogs evolve through jumps as a defensive survival response toward animal predators which stem from frightened reactions. Is fear of competitors really the right basis to set ambitious goals? Mashelkar introduced an appropriate analogy which better represented ambition. Reliance needs to perform the pole vault event rather than ordinary leaps in order to represent the magnitude of their ambitious goals.
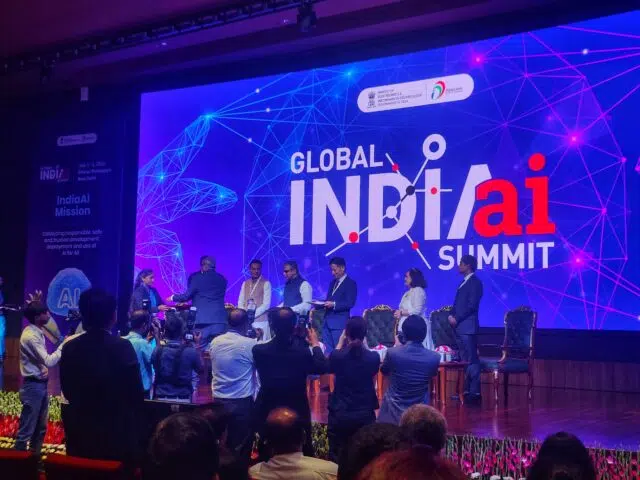
The incident with the frog continued as a precursor to the disruptive telecom entrance made by Jio during the late year of 2016. The business action had dual effects on the market by eliminating its competitors while enabling the rapid advancement of India’s digital economy to achieve first place in global mobile data usage at the same time Jio established itself as the leader with 470 million subscribers. According to Mashelkar the implementation exhibited “incredible speed and its rapid pace along with tremendous scale and sustained effects” which represented corporate pole vaulting.
Ambani’s Trajectory on AI
Ambani has focused his sights on reaching another vault even though Jio has solidified its position as India’s core digital network. Ambani aims to seize the artificial intelligence (AI) wave which businesses worldwide are riding while it sweeps through the market.
India’s AI Ecosystem is Getting Bigger Than Ever
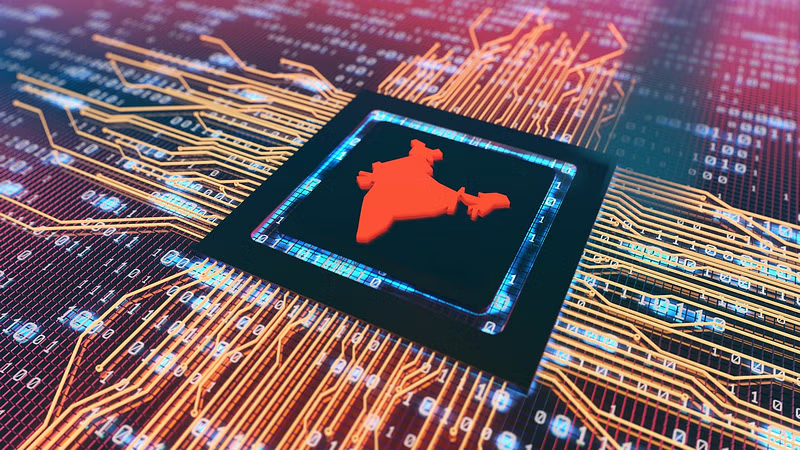
India’s AI Initiatives: The Indian Artificial Intelligence objectives transcend the scope of Reliance Industries. Nvidia and Tata Group established a partnership to develop AI infrastructure which currently outperforms all other supercomputers operating in India. The start-up sector in India secured $560 million through 25 funding rounds during 2023 as Builder.ai received $250 million through Series D funding. Enterprise-level AI solutions make up the main focus area of these start-up ventures.
India’s AI Ecosystem: Indigenous AI capabilities in the country received vigorous support from the government. The INDIAai program established its operations in 2021 by opening government data to both academics and start-ups for research and development purposes. The IndiaAI Mission has launched in 2024 to receive a budget exceeding ₹10,000 crore for building scalable computing infrastructure and establishing accessible AI technology platforms. The government implements two critical programs that involve establishing non-personal data platforms alongside utilizing Aadhaar metadata and ISRO’s earth observation database.
Challenges and Opportunities Due to AI in India
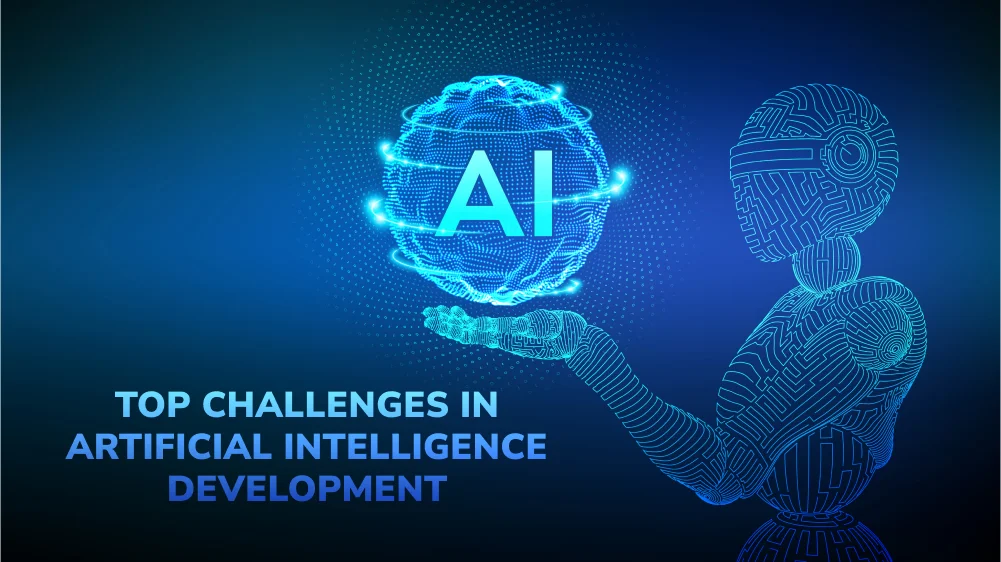
AI in India: The nation continues making strides toward AI supremacy but obstacles stand in the way of achieving full dominance. Data access quality remains essential yet difficult because privacy issues combine with unclear boundaries regarding non-personal data usage. Public information can be scraped under the Digital Personal Data Protection (DPDP) Act 2023 for data mining purposes since consent is not required while the law raises questions about ethical standards for AI technology developments.
The resolution of these problems requires start-ups to work together with organizations that hold extensive data capabilities. IBM’s “Watsonx” initiative provides large-scale foundation models which handle multiple use cases through their hybrid characteristics. Research is focused on zero-shot and few-shot learning approaches to develop multilingual natural language processing systems because Indian languages have limited training datasets.
Decentralized development of LLMs suitable for Indian languages becomes attainable through blockchain technology implementations. Through data tokenization using blockchain technology start-ups can develop affordable solutions with secure access to their digital assets and owners gain more opportunities to monetize their data assets.
AI Startups leading India in innovation
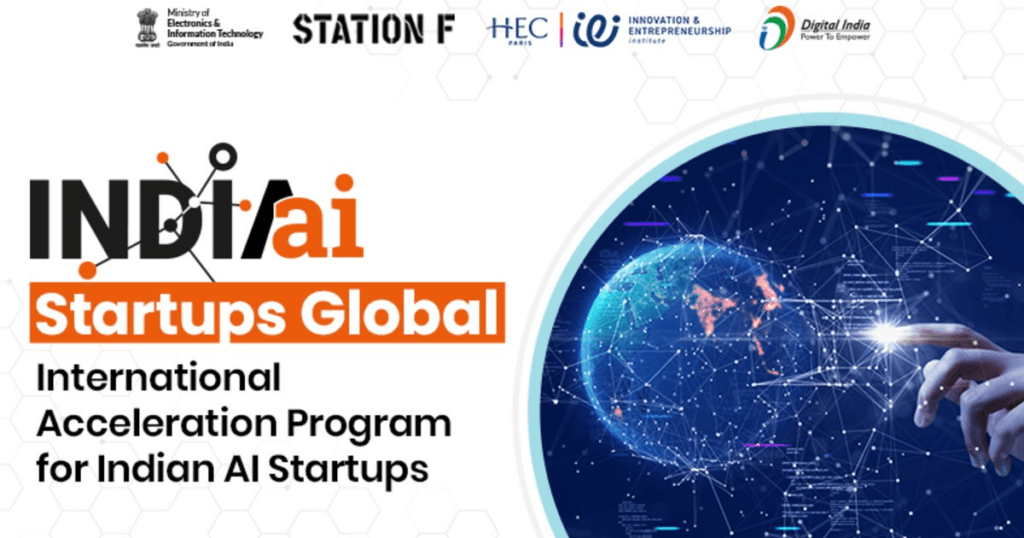
Indian startups are developing low-cost AI models tailored for local needs atop exclusive datasets. Sarvam.ai’s initiative named OpenHathi aims to produce open models and datasets at a cost that pales compared to global offerings, such as OpenAI’s GPT, in Hindi, English, and Hinglish. KissanAI has designed Dhenu 1.0, a small LLM for agriculture, from over 355,000 agricultural conversations pulled from farmers.
Cultural Inclusivity & AI in India
AI in India: Making this somewhat more efficient include automatic speech recognition and allied technology systems for live voice translation across India’s many languages. Real-time translation in many languages is about to democratize the use of technologies, and an initiative like this can bump down the latency from six seconds to one or two seconds.
Indigenous Indian AI models and language technology
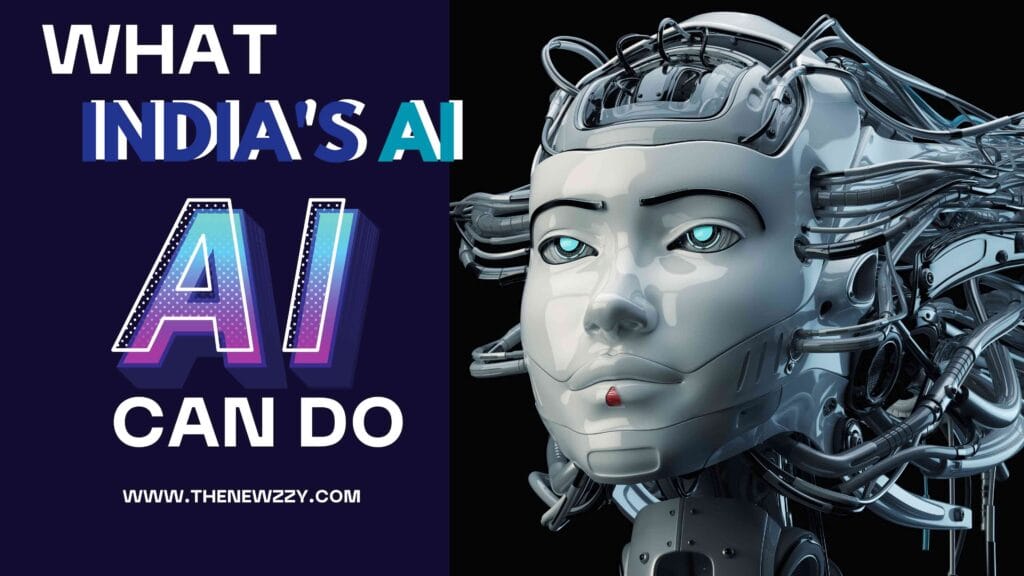
India’s AI Initiatives: The nation of India continues its quick advancement towards developing native foundational AI models. Digital India BHASHINI represents an AI-led platform for language translation that enables Indian language access to internet and digital services through voice-based interfaces. BharatGen functions as the globes first publicly funded multimodal artificial intelligence model intended to enhance public service delivery via AI-based vision and speech along with language capabilities.
AI in India: Sarvam-1 AI model exists as a specialized platform for Indian languages which assists users through language translation tasks and text summarization and content generation among others across ten major Indian languages. Users can use the open-source video transcreation platform Chitralekha to produce and modify audio transcripts which work with multiple Indic languages.
Everest 1.0 stands out as a major achievement from SML’s Hanooman because it serves 35 Indian languages and the creators aim to reach 90 languages soon.
What AI Challenges India is Facing Right Now & India’s AI Initiatives?
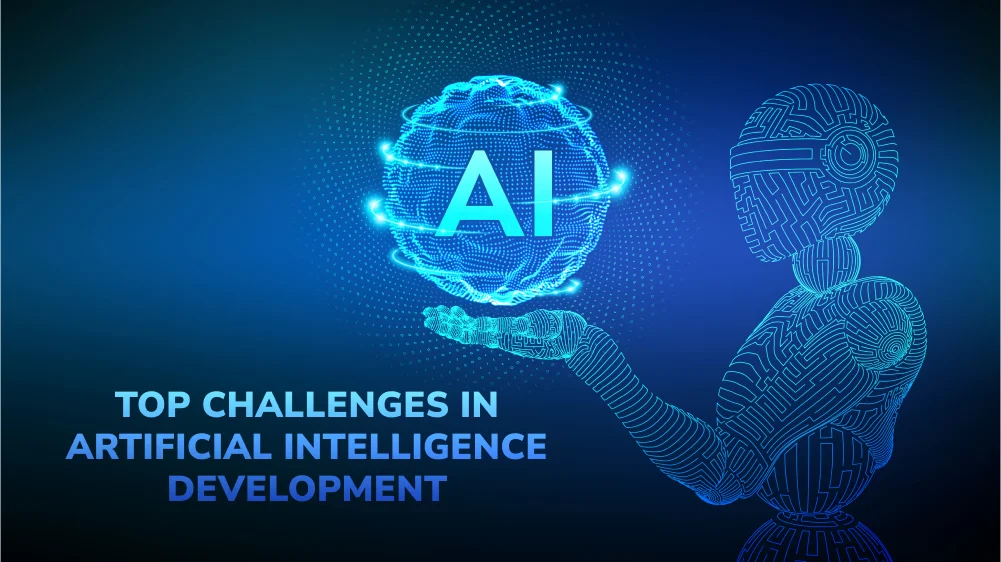
The main obstacle with large AI models is their substantial computing requirements that drive up expenses. Model growth leads to rising expenses that make them unattainable for widespread adoption throughout India specifically for inferencing tasks. The rising adoption of generative AI technologies will push computing expenses to jump 89% during the period from 2023 to 2025.
A difficulty exists because Indian applications lack sufficient ready-to-use data datasets for AI processing. Restrictive data accessibility prevents the creation of specific AI applications which address Indian market requirements.
1. Language Models
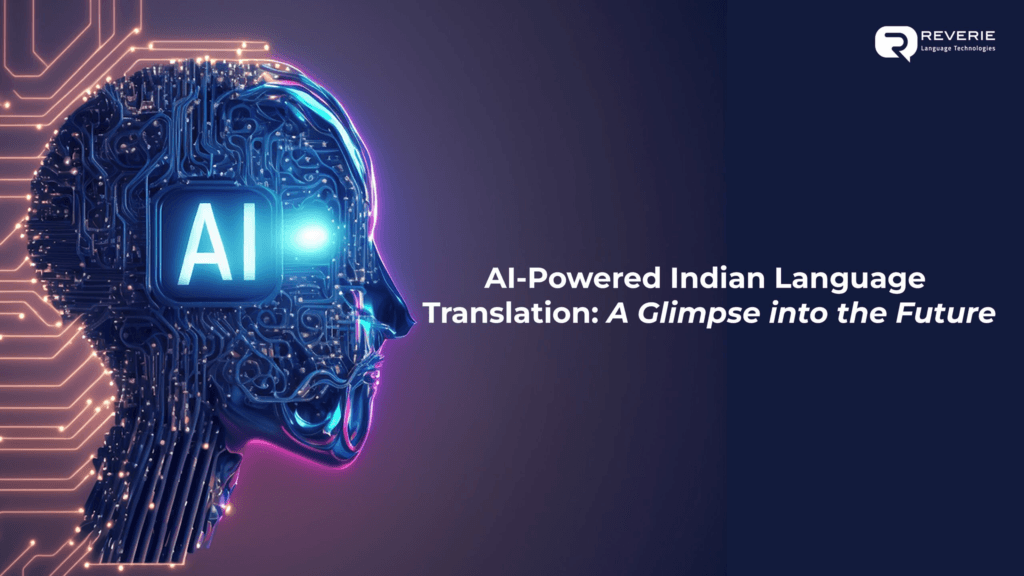
India’s AI Initiatives: The greatest challenge in indigenous language technology development is that while the needs of the community may be very much varied and cannot be assumed to be standards or similar needs among communities. For example, the linguists and technical team might have created the program with the aim of conservation or revitalisation, but the community wanted something resource access or resource sharing from such programs.
The creation of technology responsive as user has much open-ended vision by its creators. Many existing language technologies are built with visions among academics for linguistic analysis, thus disconnecting different actors’ motivations. For example, Microsoft noted that because the younger generation doesn’t go by pure Mundari when they speak, it is taking a hybrid form containing typically Bengali, Mundari, and Oriya forms.
2.Limits of Digital Content and Insufficient Data
AI in India: The minor availabilities of digital content in the Mundari script thus is de-incenting using the language as well as its script. This now means that language workers will have to go on recording observations in use of the languages and end up looking for ways to respond to them in the technology designs.
Whether through the appropriate digital spaces to create or put in people’s access, no single bias can meet the need of those who are being marginalized in such a way that they do not fall into a relevant ‘market’ for most technologies. Creation of language resources with children at Pratham had been blocked by the absence of sufficient data on children’s voices.
India’s Progress in AI
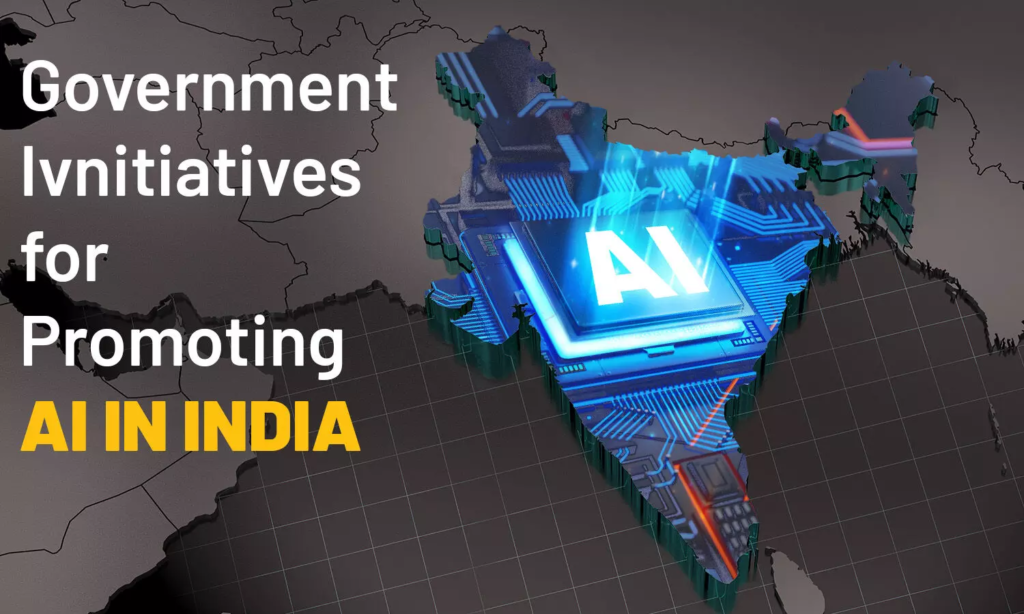
Although this last point is addressed now by work in progress towards creating libraries of digitized voice and textual data on Indian languages-an effort by INLTK, Indic NLP Library, Stanford NLP, et al.-the existing voice recognition did not properly function for children and as such, was not adequate to support the success of the program.
To deal with that, Pratham had to begin building a language corpus by children’s voice notes recorded with great attention to achieving no background noise or other extraneous sounds that would interfere with machine learning.
India’s AI Initiatives: The corpus was transcribed and annotated with volunteers, including citizen volunteers, local community members, and teachers who supervised the test. Consequently, Pratham now is able to automate the language recognition and learning process, thus enabling children to use their voices to translate and seek assistance from apps like Alexa.
Domestic Utilization
Most prevalent challenge among all six initiatives was the lack of comprehensive and sufficient linguistic data for these languages, an issue that frequently stems from inadequate investments in these languages.
The current requirement for India to operate foreign-developed AI models hinders domestic utilization of indigenous technological resources. Indian industries must obtain licenses for proprietary model GPT-4 which subjects them to external pricing structures and policy variations. External dependence on AI models causes higher expenses and enemies greater control over AI applications.
AI Computing Infrastructure
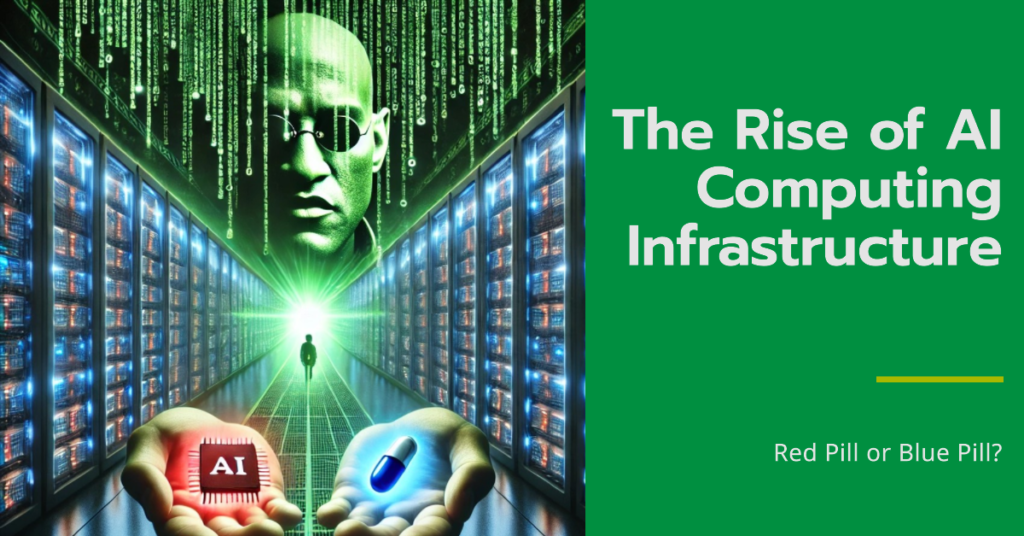
The main barrier to accessing needed AI computing infrastructure stands as infrastructure development. Indian AI solution scalability reaches its limits because the country depends on NVIDIA to supply essential AI chips and GPUs from foreign locations. The required hardware systems which need foreign import restricts both development and deployment of AI solutions.
India’s large cultural range as well as its many geographical areas and different languages create difficult barriers for designing unified AI solutions which serve the entire nation. The development of effective AI applications becomes more complicated because different accents and dialects and languages active throughout states create challenges particularly in speech recognition and translation sectors.
Efficient AI models require the ability to understand 22 official languages together with their various dialects to function effectively throughout the nation.
AI faces persistent ethical challenges partly because of its potential for abusive applications that might include deepfakes together with false information dissemination. AI responsible use and ethical standards remain a critical priority because developments happen fast and there exists potential misapplication.
How India should Advance using AI in Future?
Building Efficient Models: Preferably, India should be developing relative cost effective AI models and infrastructures to contend over high cost computing. The state is also scheduled to launch a cheap indigenously developed AI model which costs less than Rs. 100 per hour, employing a 40% subsidy to enable startups and researchers to access it. | Make Data Available: How to improve availability along with data is opening up a single point of availability for AI-ready datasets. The India AI Datasets Platform is a very essential step towards this solidified access point for making high-quality non-personal datasets available to Indian innovators around artificial intelligence. |
Sovereignty Protection: Covering the dependency on foreign models by building up a sovereign foundational AI model is an important step. The IndiaAI Mission envisages developing such a model, which will be framed according to the linguistical and cultural diversity in India. | Infrastructure Building: India must also move forward by investing in its own AI computing power to leave this limitation in computational infrastructure. The move is part of the government’s larger plan to procure 10,000 GPUs in about 18-24 months halves for boosting AI research and development. |
Inclusive Models: The plurality of India’s reality-its languages, cultures, and geographical aspects-must be poured into the solutions for AI. The AI for India 2030 initiative underlines adoption for all-inclusive and responsible AI to serve the socio-economic environment. | Ethical AI: Establishing a sound definition of ethics and formulating sound frameworks will govern ethical AI. Now, Indians have the benefit of a comprehensive integrated approach towards governance of AI by NITI Aayog’s Responsible AI for All framework. |
Read More Research Based Exclusive Content on Newzzy